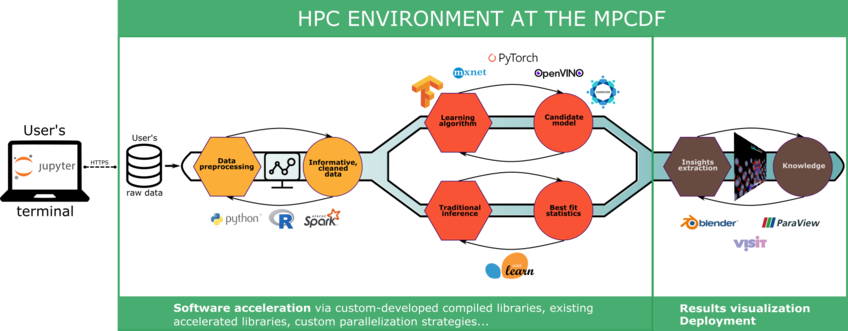
AI & Data Analytics
The MPCDF provides end-to-end solutions on HPC systems for artificial intelligence (AI), machine learning (ML) and high performance data-analytics (HPDA) for Max Planck scientists. This ranges from the integration of the infrastructure (such as Jupyter notebooks, remote visualization services, support of Apache Spark, etc.) into the HPC system, the provisioning of HPC optimized software (like various frameworks for deep learning and AI), as well as dedicated high-level project support in close collaboration with MPG research groups. Project support comprises of the consulting on state of the art techniques, of the development and optimization of data analytics (especially deep learning and AI) solutions.
Support
For dedicated project support, please contact A. Marek (HPDA).
Ongoing projects
- Development of a multiscale magnetic materials modeling suite (MaMMoS project, funded by the European Union).
- Representation learning with reinforcement learning (MPI for Human Cognitive and Brain Sciences, Leipzig)
- Measuring code flexibility in LLMs (MPI for Human Cognitive and Brain Sciences, Leipzig)
- MRI Segmentation using synthetic images (MPI for Human Cognitive and Brain Sciences, Leipzig).
- GANs for chemical structure generation (MPI-FHI, Berlin)
- Construction of a knowledge graph for the history of (socio)legal scholarship (MPI-LHLT, Frankfurt am Main)
- LLMs for automatic paper analysis (MPI for Collective Goods, Bonn)
Shortlist of completed projects
- I/O optimization and parallelization of deep learning applications for material sciences within the BIGmax collaboration (MPI for Sustainable Materials, Düsseldorf).
- MRI Reconstruction with deep learning (MPI for Human Cognitive and Brain Sciences, Leipzig).
- 3D deep learning for enhanced atom probe tomography analysis, as a chapter of the BIGmax collaboration (MPI for Sustainable Materials, Düsseldorf).
- 3D dust mapping of the Milky Way (MPI for Astronomy, Heidelberg).
- Training of a LLM to automatize the search of specific materials within the materials science literature (MPI for Sustainable Materials, Düsseldorf).
- Cell image segmentation via deep learning networks (MPI for Microstructure Physics, Leipzig).
- Solving the laplacian connectivity embedding problem in MRI imaging via the ELPA library (MPI for Human Cognitive and Brain Sciences, Leipzig).
- AI assistet recognition of nanoscale structures (MPI for Sustainable Materials, Düsseldorf).
- Integration of environmental information into thermal videos for wild animal tracking (Max Planck Institute for Animal Behavior, Konstanz).
- Deep-prior for denoising of MRI images (MPI for Human Cognitive and Brain Sciences, Leipzig).
- Next generation sequencing at scale (MPI for Biology of Ageing, Cologne).
- 2D and 3D segmentation of cellular structures (BigMax and MPI of Colloids and Interfaces, Potsdam).
- Particle track reconstruction (MPI for Physics, Munich).
- Robust classification of neuro-degenerative diseases (MPI for Human Cognitive and Brain Sciences, Leipzig).
- Dataset creation with a BigGAN (MPI for Human Cognitive and Brain Sciences, Leipzig).
- Optimizing the RoboEM application for synaptic-resolution connectomics (MPI for Brain Research, Frankfurt am Main).
- Distributed training of deep learning networks (MPI for Plasmaphysics, Garching near Munich)
- 3D segmentation of histology data (MPI for Human Cognitive and Brain Sciences, Leipzig)
People
Members of the AI & HPDA division:
- Dr. Andreas Marek (leader)
- Dr. Timoteo Colnaghi
- Dr. Piero Coronica
- Dr. David Carreto Fidalgo
- Nastassya Horlava
- Christina Winkler
Members of other divisions working partly for the AI & HPDA division
- Dr. Michele Compostella (System Operations division)
- Dr. Nicolas Fabas (Data Services division)
- Dr. John Alan Kennedy (Data Services division)
Former members of the data-analytics group
- Dr. Giuseppe di Bernardo (Industry)
- Dr. Pavel Kus (University of Prague)
- Dr. Luka Stanisic (Industry)
Talks and Training Material
- High-performance Data Analytics (HPDA) at the MPCDF. M. Rampp & A. Marek. Talk presented at DV-Treffen der Max-Planck-Institute. Göttingen, Germany, Sep 17-19, 2019.
- High-performance Data Analytics: Basic concepts of distributed deep learning. M. Rampp & A. Marek. Invited lecture at the BiGmax Big Data Summer 2019, Platja d'Aro (Spain), Sep 9-13, 2019.
Shortlist of Publications
For a complete list see: Publications by MPCDF
- Y. Wu, T. Colnaghi, A. Marek, M. Rampp et al. 3D deep learning for enhanced atom probe tomography analysis of nanoscale microstructures, Acta Materialia 278, 120280 (2024).
- T. Colnaghi, A. Marek et al., The role of AI and ML in the development of a multiscale modeling suite for sustainable magnetic materials, 1st ai4am Conference, Barcelona (2024).
- T.E. Dharmawardena, P. Coronica, T. Colnaghi et al., All-sky three-dimensional dust density and extinction Maps of the Milky Way out to 2.8 kpc, MNRAS 532, 3480 (2024).
- K.M. Dank, P. Coronica et al.,NeuroQuantify – An image analysis software for detection and quantification of neuron cells and neurite lengths using deep learning, Journal of Neuroscience Methods 411, 110273 (2024).
- Y. Li, T. Colnaghi, A. Marek, M. Rampp et al., Machine Learning-Enabled Tomographic Imaging of Chemical Short-Range Atomic Ordering, Advanced Materials, 2407564 (2024).
- Y. Li, T. Colnaghi, A. Marek, M. Rampp et al., Quantitative three-dimensional imaging of chemical short-range order via machine learning enhanced atom probe tomography, Nature Communications 14, 7410 (2023). [Link]
- Z. Rao, T. Colnaghi, A. Marek, M. Rampp, et al., Direct recognition of crystal structures via three-dimensional convolutional neural networks with high accuracy and tolerance to random displacements and missing atoms, Scripta Materialia 234, 115542 (2023). [Link]
- T.E. Dharmawardena, P. Coronica, T. Colnaghi et al., The three-dimensional structure of galactic molecular cloud complexes out to 2.5 kpc, MNRAS 519, 228–247 (2023). [Link]
- Y. Li, T. Colnaghi, A. Marek, M. Rampp et al., Convolutional neural network-assisted recognition of nanoscale L12 ordered structures in face-centred cubic alloys, Npj Comput. Mater. 7, 1-9 (2021). [Link]